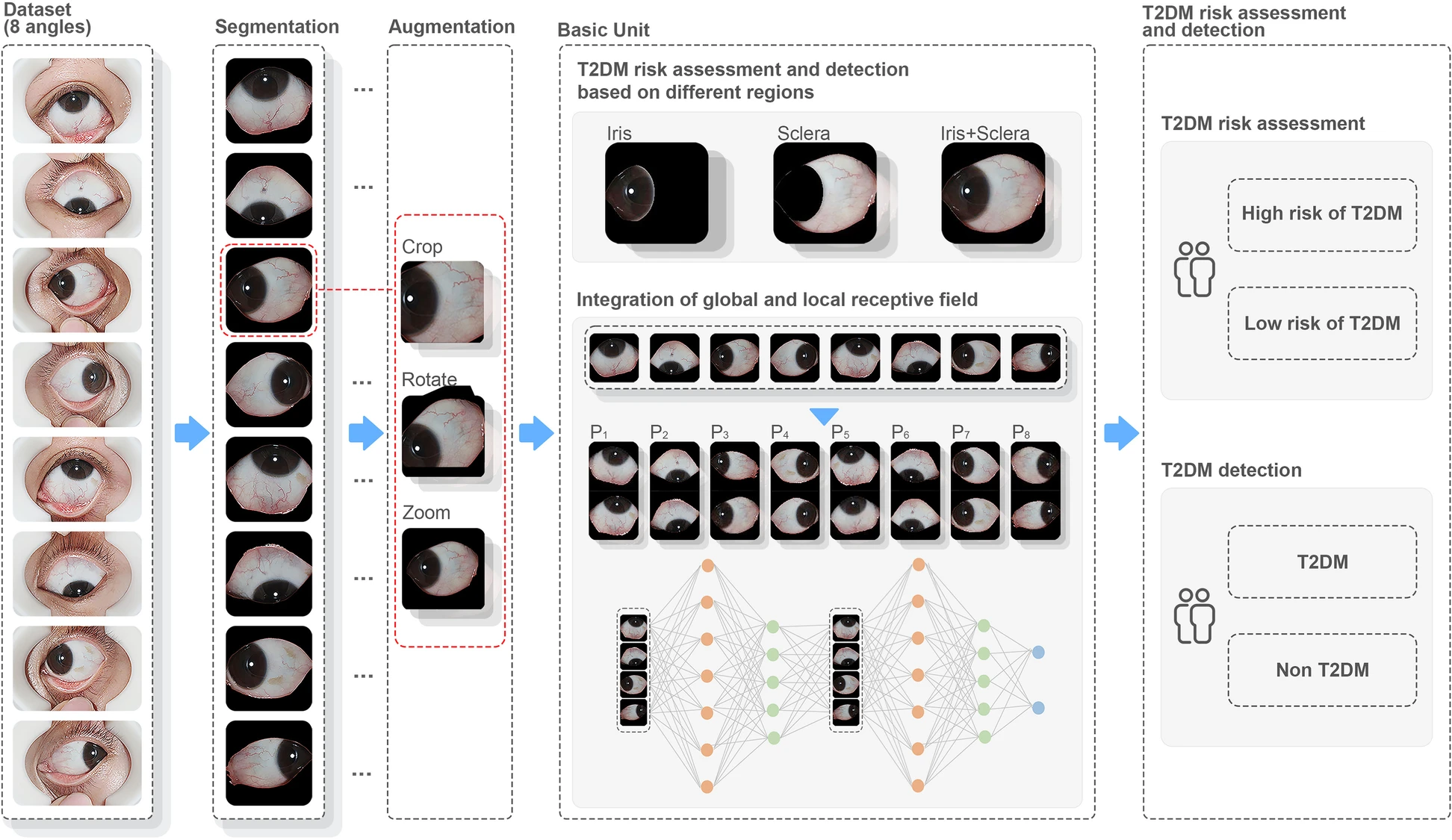
Abstract
The global prevalence of diabetes is steadily increasing, with a high percentage of patients unaware of their disease status. Screening for diabetes is of great significance in preventive medicine and may benefit from deep learning technology. In traditional Chinese medicine, specific features on the ocular surface have been explored as diagnostic indicators for systemic diseases. Here we explore the feasibility of using features from the entire ocular surface to construct deep learning models for risk assessment and detection of type 2 diabetes (T2DM). We performed an observational, multicenter study using ophthalmic images of the ocular surface to develop a deep convolutional network, OcularSurfaceNet. The deep learning system was trained and validated with a multicenter dataset of 416580 images from 67151 participants and tested independently using an additional 91422 images from 12544 participants, and can be used to identify individuals at high risk of T2DM with areas under the receiver operating characteristic curve (AUROC) of 0.89–0.92 and T2DM with AUROC of 0.70–0.82. Our study demonstrated a qualitative relationship between ocular surface images and T2DM risk level, which provided new insights for the potential utility of ocular surface images in T2DM screening. Overall, our findings suggest that the deep learning framework using ocular surface images can serve as an opportunistic screening toolkit for noninvasive and low-cost large-scale screening of the general population in risk assessment and early identification of T2DM patients.
Highlights
-
Phenotypes of ocular surface can be used for accurate, non-invasive, affordable T2DM risk assessment.
-
Ocular surface phenotypes associated with T2DM risk preliminarily elucidated by the neural network OcularSurfaceNet.
-
It has potential for generalized high-impact application in T2DM screening for large-scale population.